L'objectif du projet Artificial Creative Intelligence and Data Science (ACIDS) est de modéliser la créativité musicale en développant des modèles d'intelligence artificielle et d’apprentissage machine innovants et de fournir des outils d'exploration intuitive de la créativité. Le projet fournit une ample activité théorique, de modélisation et d’expérimentation d’outils.
L'étude de la créativité dans des situations interactives humain – IA est déterminante pour la compréhension des interactions « symbiotiques ». Le fait de disposer de modèles d'intelligence artificielle capables de démontrer des comportements créatifs pourrait donner naissance à une toute nouvelle catégorie de systèmes d'apprentissage créatif génériques.
Le temps est l'essence même de la musique, et pourtant c'est une donnée complexe, à plusieurs échelles et à plusieurs facettes. C'est pourquoi la musique doit être examinée à des granularités temporelles variables, car une multitude d'échelles de temps coexistent (de l'identité de notes individuelles jusqu'à la structure de morceaux entiers). Nous introduisons donc l'idée d'un apprentissage de la granularité temporelle profonde qui pourrait permettre de trouver non seulement les caractéristiques saillantes d'un ensemble de données, mais aussi l'échelle de temps à laquelle elles se comportent le mieux.
Nous avons par exemple récemment (projet ACTOR) mis au point le premier système de Live Orchestral Piano par apprentissage automatique de répertoires piano/orchestre, permettant de composer de la musique avec un orchestre classique en temps réel en jouant simplement sur un clavier MIDI. En observant la corrélation entre partitions de piano et orchestrations correspondantes historiques, nous pourrions déduire la connaissance spectrale des compositeurs. Les modèles probabilistes que nous étudions sont des réseaux de neurones avec des structures conditionnelles et temporelles.
ACIDS encourage un modèle interactif et centré sur l'utilisateur, où nous visons à placer l’humain au centre. Par exemple (collaboration avec les projets REACH et MERCI de l’équipe), les interactions collectives humain machine, notamment l’improvisation nous intéressent comme modèle général des interactions humaines dans lesquelles la décision, l'initiative et la coopération sont à l'œuvre, et constituent un pont d'observation et de contrôle idéal pour comprendre et modéliser l'interaction symbiotique en général.
Alors que la plupart des recherches actuelles tentent de surpasser les approches précédentes en utilisant des modèles plus complexes et plus lourds, nous encourageons le besoin d'un modèle simple et contrôlable. En outre, nous sommes convaincus que les modèles véritablement intelligents devraient pouvoir apprendre et généraliser à partir de petites quantités de données. L'une des idées clés d’ACIDS est basée sur l'hypothèse de la multiplicité. Ce concept affirme que des informations très complexes dans leur forme originale pourraient se trouver dans un espace plus simple et organisé. Nous cherchons donc à modéliser cette sémantique de haut niveau de la musique par la notion d'espaces latents. Cela pourrait permettre de comprendre les caractéristiques complexes de la musique mais aussi de produire des paramètres de contrôle compréhensibles.
Les espaces de timbre ont été utilisés pour étudier les relations entre les différents timbres instrumentaux, sur la base de notations perceptuelles. Cependant, ils ne permettent qu'une interprétation limitée, aucune capacité de génération et aucune généralisation. Nous étudions dans ACIDS les auto-encodeurs variationnels (VAE) qui peuvent pallier ces limitations, en régularisant leur espace latent pendant la formation afin de s'assurer que l'espace latent de l'audio suit la même topologie que celle de l'espace de timbre perceptif. Ainsi, nous faisons le pont entre l'analyse, la perception et la synthèse audio en un seul système. Les synthétiseurs de son sont omniprésents dans la musique et ils définissent même maintenant entièrement de nouveaux genres musicaux. Cependant, leur complexité et leurs ensembles de paramètres les rendent difficiles à maîtriser. Nous avons créé un modèle probabiliste génératif innovant qui apprend une correspondance inversible entre un espace auditif latent continu des capacités audio d'un synthétiseur et l'espace de ses paramètres. Nous abordons cette tâche en utilisant des auto-encodeurs variationnels et en normalisant les flux. Grâce à ce nouveau modèle d'apprentissage, nous pouvons apprendre les principales macro-commandes d'un synthétiseur, ce qui nous permet de voyager à travers sa multitude de sons organisés, d'effectuer des inférences de paramètres à partir de l'audio pour contrôler le synthétiseur avec notre voix, et même d'aborder l'apprentissage de la dimension sémantique où nous trouvons comment les commandes s'adaptent à des concepts sémantiques donnés, le tout dans un modèle unique.
Les travaux d’ACIDS sont régulièrement utilisés en création contemporaine, par exemple dans la production de la voix d’un chanteur d'opéra synthétique (La fabrique des monstres, Ghisi / Peyret) ou des collaborations avec le compositeur Alexandre Schubert sur apprentissage et captation gestuelle.
Équipe Ircam : Représentations musicales
- ACIDS
- ACIDS
- ACIDS
Détails du projet
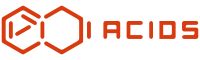