Dans le cadre du projet HOULE, nous proposons des techniques originales d’apprentissage non supervisé pour résoudre le problème de la structuration des scènes auditives (Computational Auditory Scene Analysis : CASA), problème consistant à identifier et décrire les objets sonores constituant une scène. CASA est un domaine très actif du traitement du son ayant de nombreuses applications. Les points bloquant les méthodes actuelles sont :
- Ne pouvant faire d’hypothèse sur la nature des objets traités, on ne peut les modéliser a priori ;
- Les objets mélangés dans la scène ne peuvent être observés en isolation ;
- Leur structure est gouvernée par de nombreuses relations dont la priorité est difficile à établir.
Les caractéristiques dont nous tirons parti pour notre approche sont, l’organisation hiérarchique des scènes audio (atomes rassemblés en objets qui sont des instances de classes comme « La4 de piano », elle-même un exemple de « Note de piano »), et la redondance présente à tous les niveaux de cette hiérarchie. Cette redondance nous permet d’identifier des motifs récurrents sur lesquels fonder une représentation riche et robuste.
À cette nouvelle approche de la représentation de scènes audio correspond un algorithme d’apprentissage non supervisé spécialement conçu pour traiter ces données. Le système est structuré en deux composants : le Regroupement Multi-Niveaux (RMN) opère la structuration des données, tandis que le « Superviseur » (module d’adaptation réflexive) incarne l’aspect « apprentissage » en optimisant à la volée le fonctionnement de RMN en référence à une mémoire d’exécutions passées.
L’originalité de notre proposition tient dans son détachement des approches traditionnelles à CASA, en commençant par le paradigme de représentation des scènes et objets. L’innovation est principalement présente dans les méthodes d’apprentissage non supervisé que nous allons développer, dont les applications dépassent largement le cadre de CASA.
Détails du projet
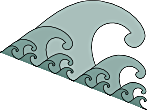